Mitutoyo has announced the release of its new AI Inspect Software to its overall lineup of software solutions.
Intended for use in determining defects in multiple applications, Mitutoyo’s AI INSPECT is a standalone software product designed to assist customers with next generation visual defect analysis.
Visual defect detection has traditionally been a costly and time-consuming operation. Now, by leveraging the immense power of artificial intelligence and machine learning, AI INSPECT from Mitutoyo allows everyday users to create simple, world-class defect detection solutions for visual inspection.
AI INSPECT software uses modern deep learning convolutional networks to learn the visual differences between normal and defect pixels in any set of correlated images.
Superior Image Processing – Provides robust compensation for brightness variation and out of focus inspection conditions.
Highest Accuracy – Industry-leading pixel level defect detection provides the highest detection rates and lowest false positives.
High Throughput – Supports up to 5.2MPx images for high throughput, large area, or high resolution inspection.
Minimal Training – Operators can be self-trained through a 25-minute demonstration video and embedded help files.
The multi-modular design AI Inspect Software features two distinct modules – Training Module and Runtime Module.
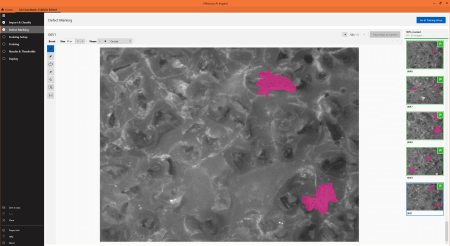
Training Module:
The main module of the software contains functionality to allow users to develop inspection routines for automated implementation of defect detection using image sets. Methodologies include: Project Management of Image Sets: Importation, organization, and storage of user defined inspection projects.
Import and Classification:
Integrated tool sets and techniques for loading and classifying both normal (good) and defect (bad) image sets.
Defect Marking:
A simplified user interface for the operator to mark-up/highlight defects on images.
Training Setup:
Allows the operator to perform a wide range of tasks, including training mode with testing and analyzation and optimization of defect detection models. Additionally, the image augmentation section allows operators to apply advanced techniques to the inspection models such as brightness, noise and focus to reduce the influence of typical image aberrational factors that may influence the accuracy of training model.
Training:
Provides status for the operator to visualize the progress and success of the training sequence.
Results and Thresholds:
A graphical GUI allows the operator to review number of predictions related to the trained model. These include image classifications and predictions, pixel predictions and defect size thresholds, and the overall model prediction of normal, uncertain, defect and misclassified trained model components.
Deploy:
Publish the model for Inspection. Additionally, the ability to publish the model and provide a unique identifier completes the entire deployment process.
Runtime Module:
The Runtime Module allows trained AI module to be incorporated into an inspection system. Included in the Runtime module model is the inference testing application that allows review of the AI model for sample testing on new images.